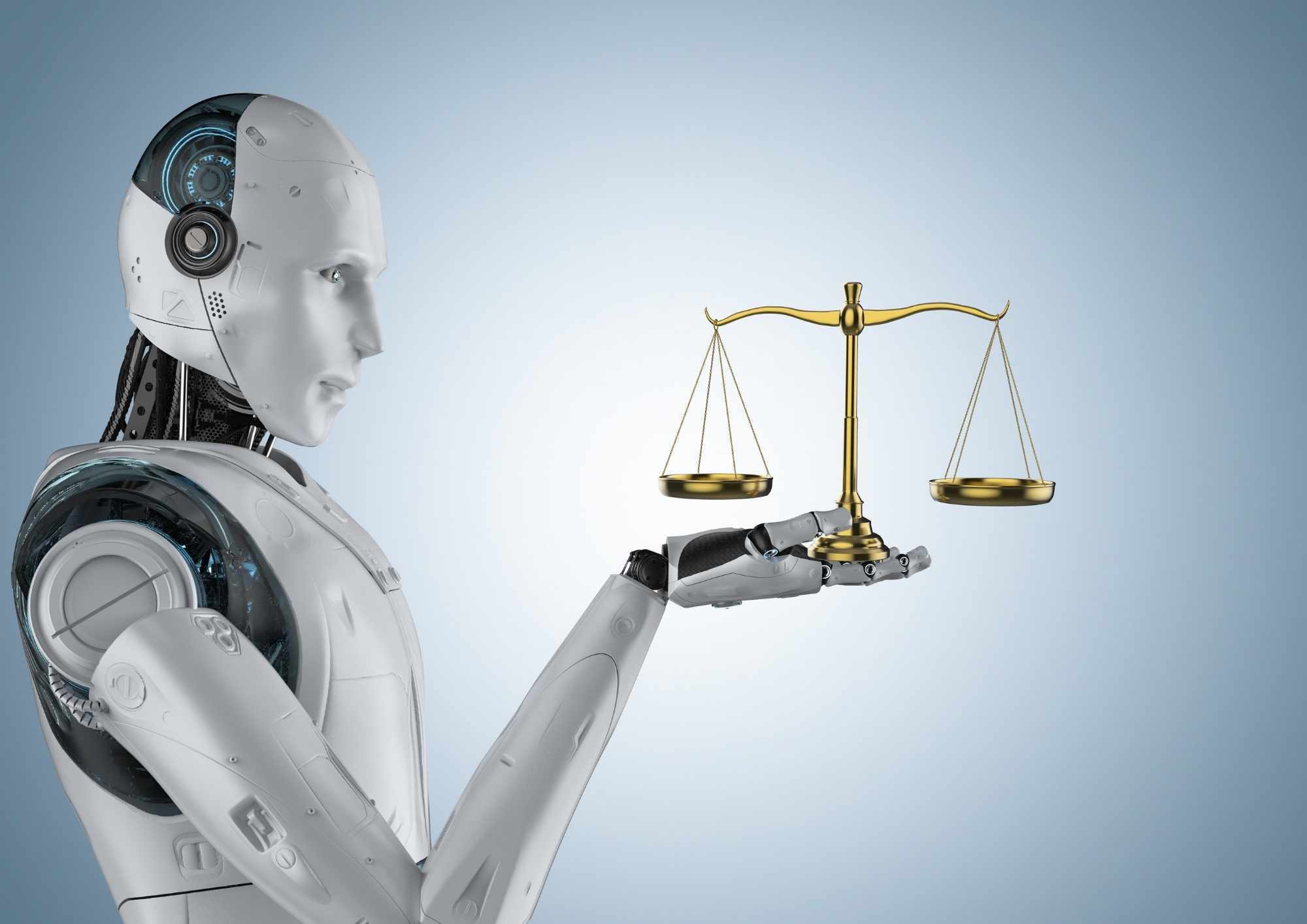
Strategies for Achieving Fair AI by Reducing Bias
Introduction:
AI systems are
embedded in everyday life, from the recommendations we receive on our favorite
streaming platforms to the automated decision-making processes in healthcare,
finance, and even criminal justice. As AI's influence extends far and wide, so does
its potential to shape and, regrettably, misshape our world.
One of the most pressing concerns accompanying the rise of AI is the issue of bias. While AI is often celebrated for its impartiality and objectivity, it can paradoxically harbor the very biases it was meant to eliminate. These biases, deeply rooted in historical data, can find their way into the algorithms, influencing the outcomes, recommendations, and decisions made by AI systems.
The consequences of biased AI are profound. They affect the lives of individuals, influence economic and social disparities, and impact societal perceptions and values. The path to achieving fair AI, free from bias, is paved with challenges, but it is a journey that must be undertaken to ensure that AI systems are equitable, accountable, and just.
This blog delves into the strategies and approaches that can be adopted to reduce bias in AI, moving us closer to the goal of achieving fair and unbiased AI systems. We will explore various techniques and best practices that can be implemented, shedding light on the steps that can be taken to mitigate AI bias effectively. Understanding these strategies is critical, not only for the developers of AI systems but for all those whose lives are increasingly intertwined with the algorithms that power our modern world.
1. Diverse and Representative Data
Achieving fair AI begins with the data used to train machine learning models. If the training data is skewed or unrepresentative of the real-world population, the AI system can inherit these biases. To mitigate this, it's crucial to collect diverse and representative data that includes various demographic groups and viewpoints. This approach allows AI models to learn from a more comprehensive and unbiased dataset.
2. Preprocessing and Data Cleaning
Data preprocessing plays a vital role in reducing bias. It involves cleaning and preparing the data before it's used to train AI models. During this stage, you can identify and address potential biases in the data. This may include removing or correcting biased data points, ensuring balanced sample sizes, and identifying and addressing outliers.
3. Algorithmic Fairness
Incorporating fairness into the algorithms themselves is another essential strategy. Algorithmic fairness ensures that the model's predictions or decisions do not discriminate against any specific group. Various mathematical techniques, such as reweighing training examples or modifying objective functions, can be used to achieve algorithmic fairness.
4. Continuous Monitoring and Auditing
Achieving fair AI is an ongoing process. Continuous monitoring and auditing of AI systems are essential to identify and rectify biases that may emerge over time. This involves setting up regular assessments, examining the outcomes of AI decisions, and making adjustments as needed.
5. Transparency and Explainability
Transparency is key to ensuring that AI systems are fair and unbiased. Being able to explain how an AI model reaches a decision is crucial. Explainable AI (XAI) techniques allow for greater visibility into the decision-making process, helping to identify and correct bias more effectively.
6. Diverse Development Teams
The composition of the teams that develop AI systems matters. Diverse teams are more likely to recognize and mitigate bias effectively. Different perspectives and experiences can help identify potential biases in the data, algorithms, and decisions.
7. Ethical Guidelines and Regulations
Ethical guidelines and regulations provide a framework for building fair AI. Many organizations and governments are developing guidelines and regulations to address bias in AI. Familiarity with and adherence to these standards can help organizations create more responsible AI systems.
Conclusion
Bias in AI is a complex issue, but strategies for achieving fair AI are within reach. By understanding AI bias, collecting diverse data, preprocessing and cleaning data, implementing algorithmic fairness, continuous monitoring, promoting transparency, fostering diversity in development teams, and adhering to ethical guidelines, we can work towards AI systems that are more equitable and less prone to bias. These strategies are essential to ensure AI's benefits are realized by all, without discrimination or unfairness.
Full Stack Development Courses in Different Cities
- Srinagar
- Bangalore
- Gujarat
- Haryana
- Punjab
- Delhi
- Chandigarh
- Maharashtra
- Tamil Nadu
- Telangana
- Ahmedabad
- Jaipur
- Indore
- Hyderabad
- Mumbai
- Agartala
- Agra
- Allahabad
- Amritsar
- Aurangabad
- Bhopal
- Bhubaneswar
- Chennai
- Coimbatore
- Dehradun
- Dhanbad
- Dharwad
- Faridabad
- Gandhinagar
- Ghaziabad
- Gurgaon
- Guwahati
- Gwalior
- Howrah
- Jabalpur
- Jammu
- Jodhpur
- Kanpur
- Kolkata
- Kota
- Lucknow
- Ludhiana
- Noida
- Patna
- Pondicherry
- Pune